Data is the new gold. It is an essential ingredient in making informed business decisions, and it can also help you identify opportunities for growth and optimization.
However, collecting and preparing data for analysis can be time-consuming and overwhelming. In this blog, we will discuss what data collection and preparation are, why it’s important to do them efficiently, and how you can optimize your analytics process.
We’ll provide you with some best practices for efficient data collection, including gathering relevant data, assessing data quality, cleansing and validating data, transforming and enriching data, and storing data in an efficient manner.
We’ll also talk about self-service analytics tools that you can use to make your analytics process more efficient. Finally, we’ll touch on the future of efficient analytics and what trends we’re seeing in the industry.

What is Data Collection and Preparation?
Data Collection and Preparation is the process of gathering, organizing, and cleaning data for analysis. It involves identifying relevant sources and selecting appropriate methods, ensuring consistency and accuracy before the analysis can start.
Accurate Data Collection and Preparation can lead to more effective data analysis.
Benefits of Efficient Analytics
Efficient analytics not only saves time and resources in data collection and preparation but also enables businesses to make informed decisions by identifying patterns and trends.
Streamlining analytics processes leads to faster insights, resulting in operational improvements for businesses.
Raw data collected from various sources can be transformed into meaningful datasets with the right data preparation steps, including cleaning, integration, and validation.
Visualizations created using statistical analysis algorithms can help both research teams and business users gain a deeper understanding of incomplete data with missing values.
Analytics in Data Collection
Efficient analytics starts with collecting the right data through tools such as surveys, questionnaires, and interviews.
It involves cleaning your datasets of incomplete data and missing values that affect the accuracy of your statistical analysis. Organizing raw data in a structured manner enables business users and data scientists to save time-consuming tasks such as integrating different sources.
Analytics in Data Collection also involves using visualization techniques such as charts or graphs to draw insights from the information collected. Following best practices such as these ensures that you have high-quality datasets for analysis.
1. Gather Relevant Data
Efficient data collection and preparation involve gathering relevant data by identifying the metrics and variables required for analysis. Data scientists use various data sources such as surveys, customer feedback, and social media analytics to collect raw datasets. Implementing best practices like validation checks ensures accurate and reliable analytics.
Organizing the collected information in a structured manner facilitates quicker statistical analysis that helps solve complex business problems. Visualization techniques like charts and graphs enhance decision-making capabilities from incomplete or missing values.
Notably improving analytics with algorithms help extract valuable insights.
2. Assess Data Quality
Quality assessment is an indispensable part of the Data Collection and Preparation process since it ensures reliable and accurate results.
Quality issues like incomplete datasets, missing values, and inconsistencies can lead to misleading insights. Collecting relevant data from trustworthy sources is not enough; one must also perform an extensive cleaning process before analysis.
Various methods such as visual inspection or statistical algorithms could be used for assessing data quality. By improving the quality of your dataset during preparation steps like transformation and integration, you can gain deeper insights into your business users’ needs.
3. Cleanse and Validate Data
When preparing for data analysis, it’s essential to ensure the accuracy of datasets by removing inaccurate or irrelevant information.
To achieve this, various data validation methods such as visual inspection and statistical analysis should be employed. Additionally, automated tools can help remove time-consuming manual efforts involved in identifying missing values or incomplete data.
By following the best practices of the data preparation process, both business users and research teams can efficiently collect and transform raw data into useful insights that enable informed decision-making.
4. Transform and Enrich Data
To ensure efficient analysis of datasets, it’s important to collect the right data by following best practices for data collection.
Data collection can be a time-consuming process involving the use of algorithms and statistical analysis to gather complete and accurate information from various data sources.
Once you have collected the necessary data, you must assess its quality before transforming and enriching it.
This involves cleaning and formatting raw data to make it usable for analysis by removing duplicate or irrelevant data, filling in missing values, and adding relevant information using tools like visualization techniques.
Finally, storing this transformed dataset in an efficient manner becomes essential for quicker access by business users or the research team.
5. Store Data in an Efficient Manner
Efficient analysis of data requires proper storage techniques such as using a database management system and regular backups.
Ensure that your data is organized logically with a consistent naming convention. Data storage is fundamental to the success of any research team.
By employing best practices in data storage and understanding how to integrate datasets, businesses can provide their data scientists and business users with quick access to the right data for visualization, algorithms, statistical analysis, and more.
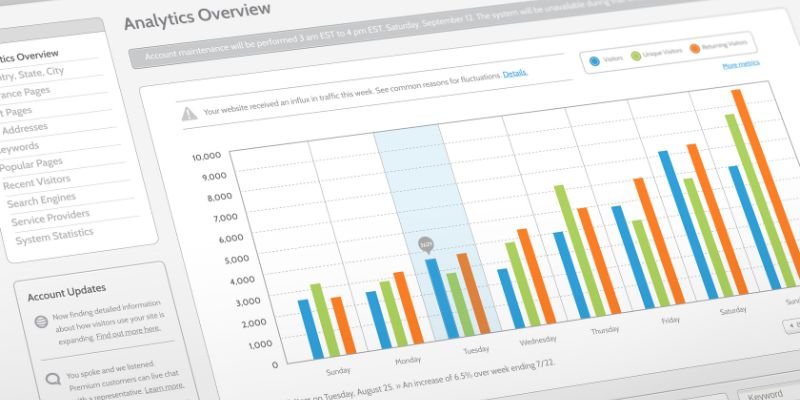
Self-Service Analytics Tools
Non-technical users can leverage self-service analytics tools to analyze and visualize their datasets for faster insights.
However, proper training is crucial for accurate data interpretation. The process of gathering relevant data requires diligent effort to avoid incomplete or irrelevant information.
Assessing the quality of collected data can be time-consuming but important to ensure reliable results.
Cleaning and validating raw data is crucial prior to transformation for statistical analysis. Efficient storage using consistent naming conventions ensures easy access later on. Self-service analytics tools complement the work of experts in fields such as data science and research teams.
Future of Efficient Analytics
In today’s world of data science and analysis, efficient analytics play a crucial role in decision-making processes.
The right data collection, and preparation steps, and utilizing automation tools for collecting raw data can save time-consuming manual processing.
Cleaning, transforming, and integrating data from multiple sources into datasets is fundamental for effective statistical analysis. Self-service tools enable business users or research teams to visualize these processes faster.
To stay ahead of your competition in this field, it’s important to use algorithms and embrace machine learning technologies while considering any ethical implications surrounding your data sources.

Frequently Asked Questions
What are some best practices for organizing and storing data for analysis?
Effective data organization and storage involve consistent naming conventions, recording data sources and transformations, secure storage with backups, and regular cleaning and updating. These practices ensure easy accessibility, accuracy, relevance, and protection against data loss.
Are there any common mistakes to avoid when collecting or preparing data for analysis?
When collecting data, avoid errors such as selecting an inadequate sample size and neglecting data quality. Preparing data for analysis requires standardizing variables and addressing missing values properly. Documenting the process ensures reproducibility. Consulting with a statistician or data expert can prevent mistakes and expedite analysis.
How can I ensure the quality and accuracy of my data before analyzing it?
To ensure accurate data analysis, define your research question or hypothesis and collect data from reliable sources using trustworthy methods. Check for errors, inconsistencies, and outliers in your data set, and conduct a pilot test or independent sample validation to confirm accuracy.
What are some tools or software that can streamline the data collection and preparation process?
There are various tools and software available to simplify the data collection and preparation process. Excel, Python, R, and SQL are common tools for data collection. For automated web scraping, Scrapy or Beautiful Soup is useful.
Trifacta or Talend can aid in data cleaning and transformation, while cloud-based platforms like Google Cloud or AWS offer scalable storage and processing options.
Here are some posts that are related
Choosing the Right Data Analysis Tool
Conclusion
Data collection and preparation are the foundation of efficient analytics. Proper data collection, cleansing, validation, transformation, and storage can help you extract meaningful insights from large datasets.
The benefits of efficient analytics are numerous, including better decision-making, increased productivity, and improved competitiveness.
Self-service analytics tools provide a powerful way to empower business users to explore data themselves without relying on IT or data science teams. Looking for more insights on how to make the most out of your data? Check out our blog on advanced data analysis techniques.